Global bacteria optimization meta-heuristic: Performance analysis and application to shop scheduling problems
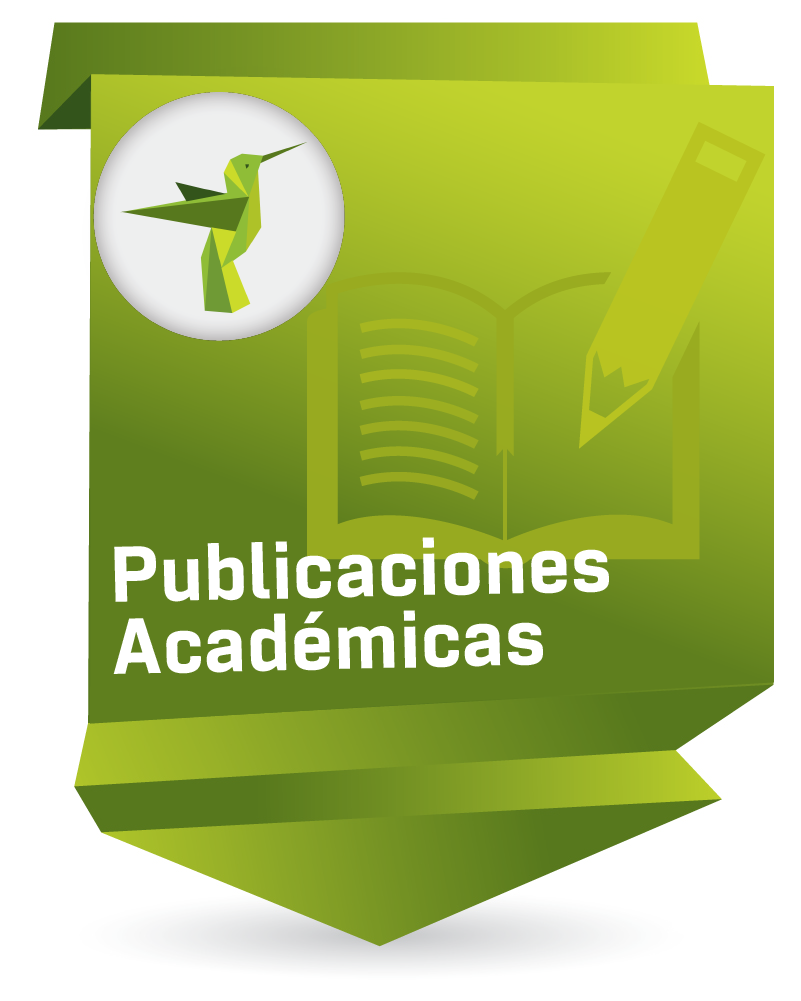
Enlaces del Item
URI: http://hdl.handle.net/10818/60183Visitar enlace: https://www.scopus.com/inward/ ...
DOI: 10.4018/978-1-61350-086-6.ch009
Compartir
Estadísticas
Ver Estadísticas de usoCatalogación bibliográfica
Mostrar el registro completo del ítemFecha
2011Resumen
A large number of real-life optimization problems in economics and business are complex and difficult to solve. Hence, using approximate algorithms is a very good alternative to solve this class of problems. Meta-heuristics solution procedures represent general approximate algorithms applicable to a large variety of optimization problems. Most of the meta-heuristics mimic natural metaphors to solve complex optimization problems. This chapter presents a novel procedure based on Bacterial Phototaxis, called Global Bacteria Optimization (GBO) algorithm, to solve combinatorial optimization problems. The algorithm emulates the movement of an organism in response to stimulus from light. The effectiveness of the proposed meta-heuristic algorithm is first compared with the well-known meta-heuristic MOEA (Multi-Objective Evolutionary Algorithm) using mathematical functions. The performance of GBO is also analyzed by solving some single- and multi-objective classical jobshop scheduling problems against state-of-the-art algorithms. Experimental results on well-known instances show that GBO algorithm performs very well and even outperforms existing meta-heuristics in terms of computational time and quality of solution. © 2012, IGI Global.
Ubicación
Hybrid Algorithms for Service, Computing and Manufacturing Systems: Routing and Scheduling Solutions p. 178-194