DARIO: Differentiable vision transformer pruning with low-cost proxies
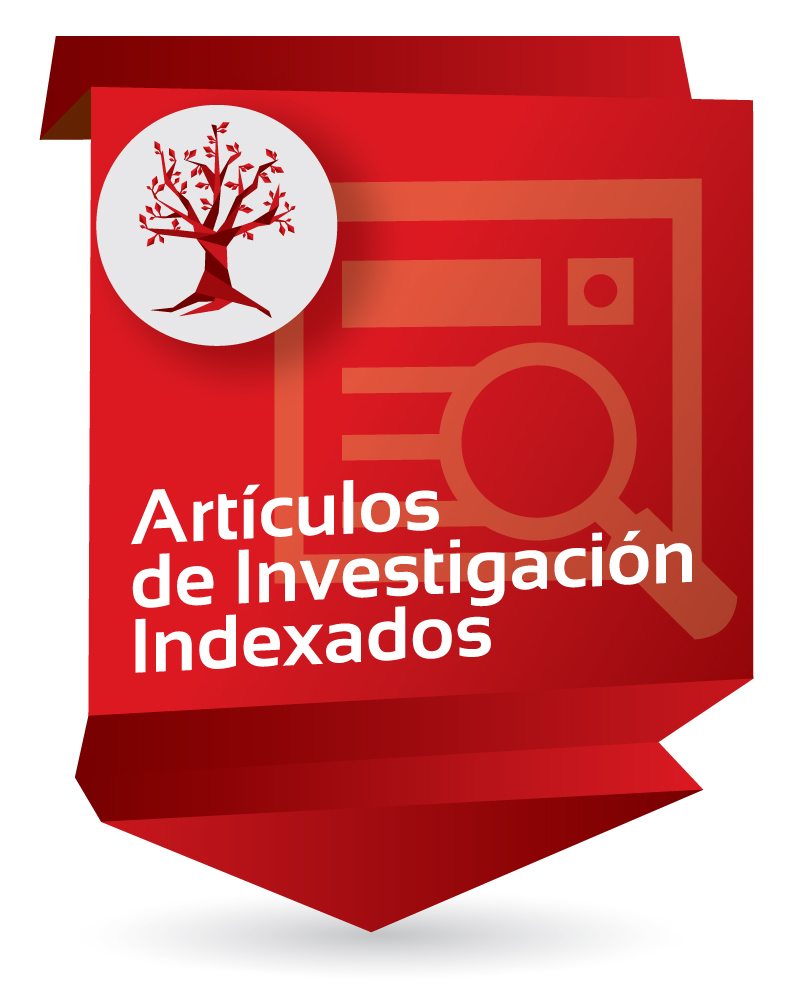
Item Links
URI: http://hdl.handle.net/10818/63362Visitar enlace: https://www.scopus.com/inward/ ...
DOI: 10.1109/JSTSP.2024.3501685
Compartir
Statistics
View Usage StatisticsBibliographic cataloging
Show full item recordDate
2024Abstract
Transformer models have gained popularity for their exceptional performance. However, these models still face the challenge of high inference latency. To improve the computational efficiency of such models, we propose a novel differentiable pruning method called DARIO (DifferentiAble vision transformer pRunIng with low-cost prOxies). Our approach involves optimizing a set of gating parameters using differentiable, data-agnostic, scale-invariant, and low-cost performance proxies. DARIO is a data-agnostic pruning method, it does not need any classification heads during pruning. We evaluated DARIO on two popular state-of-the-art pre-trained ViT models, including both large (MAE-ViT) and small (MobileViT) sizes. Extensive experiments conducted across 40 diverse datasets demonstrated the effectiveness and efficiency of our DARIO method. DARIO not only significantly improves inference efficiency on modern hardware but also excels in preserving accuracy. Notably, DARIO has even achieved an increase in accuracy on MobileViT, despite only fine-tuning the last block and the classification head. © 2007-2012 IEEE.
Ubication
IEEE Journal on Selected Topics in Signal Processing
Collections to which it belong
- Facultad de Ingeniería [547]