Learning Curve Extrapolation Methods Across Extrapolation Settings
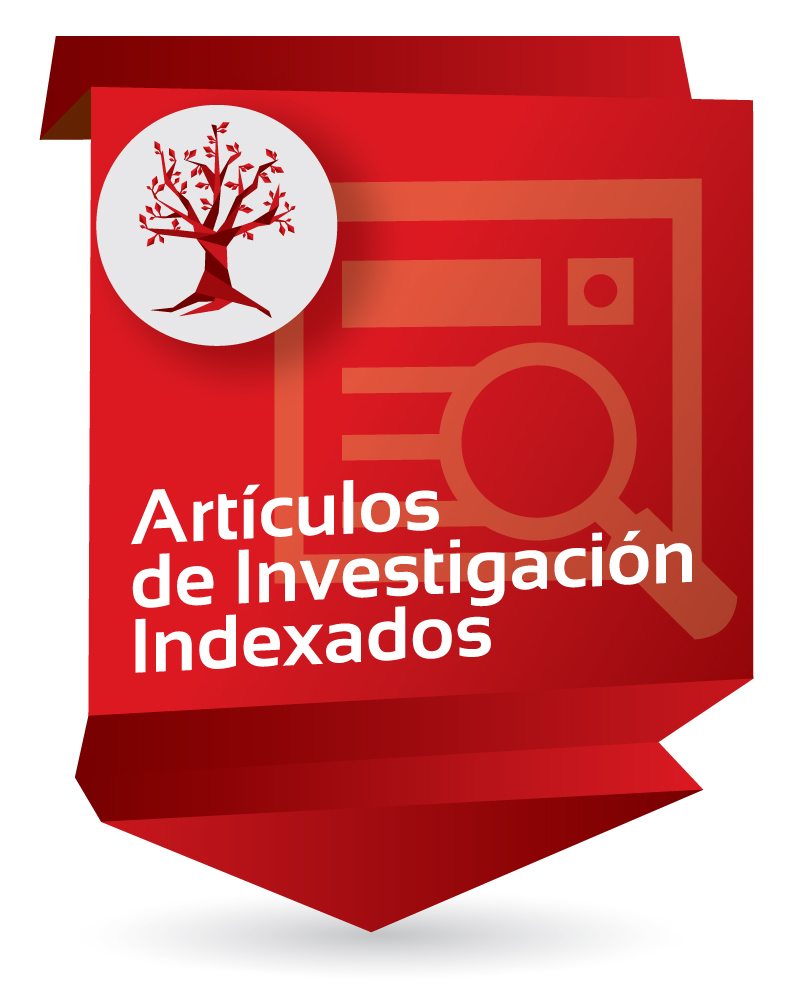
Item Links
URI: http://hdl.handle.net/10818/62756Visitar enlace: https://www.scopus.com/inward/ ...
ISSN: 3029743
DOI: 10.1007/978-3-031-58553-1_12
Compartir
Statistics
View Usage StatisticsBibliographic cataloging
Show full item recordDate
2024Abstract
Learning curves are important for decision-making in supervised machine learning. They show how the performance of a machine learning model develops over a given resource. In this work, we consider learning curves that describe the performance of a machine learning model as a function of the number of data points used for training. It is often useful to extrapolate learning curves, which can be done by fitting a parametric model based on the observed values, or by using an extrapolation model trained on learning curves from similar datasets. We perform an extensive analysis comparing these two methods with different observations and prediction objectives. Depending on the setting, different extrapolation methods perform best. When a small number of initial segments of the learning curve have been observed we find that it is better to rely on learning curves from similar datasets. Once more observations have been made, a parametric model, or just the last observation, should be used. Moreover, using a parametric model is mostly useful when the exact value of the final performance itself is of interest. © The Author(s), under exclusive license to Springer Nature Switzerland AG 2024.
Ubication
Lecture Notes in Computer Science (including subseries Lecture Notes in Artificial Intelligence and Lecture Notes in Bioinformatics) Vol. 14642 LNCS
Collections to which it belong
- Facultad de Ingeniería [547]