Missing-value imputation using the robust singular-value decomposition: Proposals and numerical evaluation
Imputación de valores faltantes usando la descomposición robusta en valores singulares: Propuestas y evaluación numérica
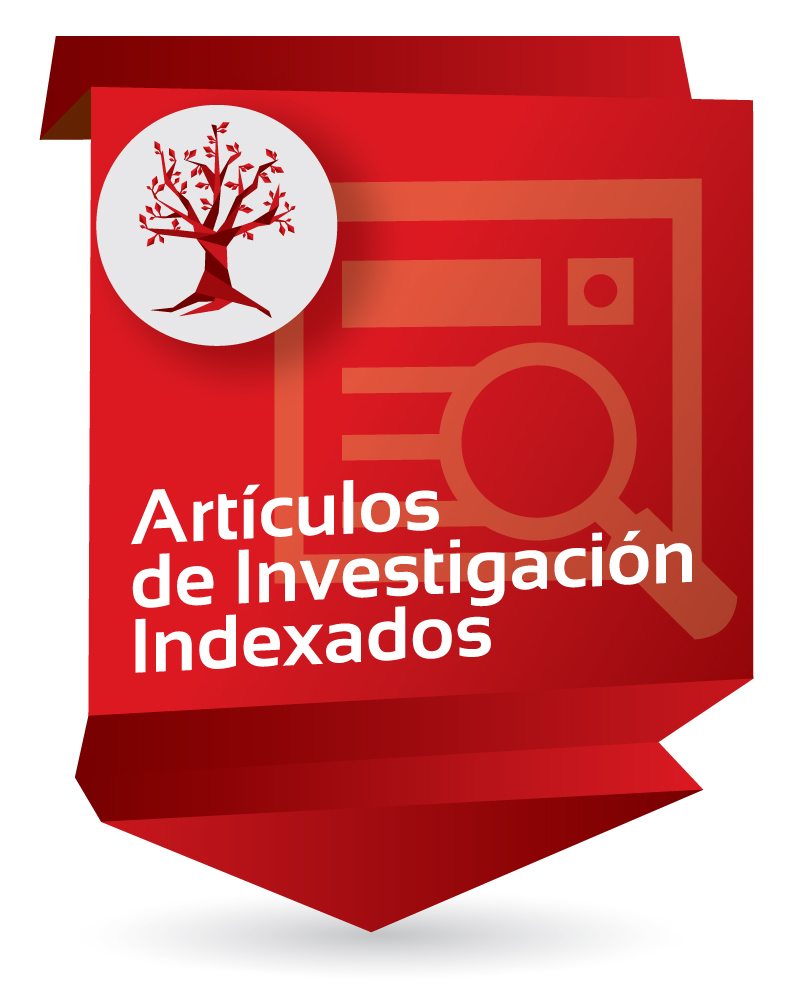
Item Links
URI: http://hdl.handle.net/10818/51122Visitar enlace: https://acsess.onlinelibrary.w ...
DOI: 10.1002/csc2.20508
Compartir
Statistics
View Usage StatisticsBibliographic cataloging
Show full item recordDate
26/03/2021Abstract
A common problem in the analysis of data from multi-environment trials is imbalance caused by missing observations. To get around this problem, Yan proposed a method for imputing the missing values based on the singular-value decomposition (SVD) of a matrix. However, this SVD can be affected by outliers and produce low quality imputations. In this article, we propose four extensions of the Yan method that are resistant to outliers, replacing the standard SVD method with four robust SVD extensions. We evaluate these methods, using exclusively numerical criteria in a simulation study and in a cross-validation study based on real data. We conclude that in the presence of outliers, the standard SVD method should not be used; instead, the best alternatives are the robust SVD methods based on sub-sampling when the percentage of contamination is less than 2% following a completely random missing data mechanism. In any other case, methods that either minimize the L2 norm or that involve L1 regressions are preferable.
Ubication
Crop Science, 61(5)
Collections to which it belong
- Facultad de Ingeniería [547]